As marketers we could learn a lot from ants.
They don’t attend conferences, have multi-million dollar budgets or get pitched by the latest AI-based tech vendors. Yet over millennia they’ve figured out a radically efficient solution to an important and complex problem – how best to find food to sustain the colony.
This is no easy task. The first ant leaving the colony walks around in a random pattern. It’s likely he (foraging ants are always male) doesn’t find food, so he’ll return back to the colony exhausted. It’s not a completely wasted effort however, he (and every other ant behind him) will leave behind a pheromone trail that attracts other ants.
Over the course of time and thousands of individual ant voyages, food will (likely) be found. Ants that do find food will return immediately back to the colony. Other ants will follow this trail and, because pheromone trails evaporate over time, they’re most likely to follow the shortest, most traveled (highest density) path.
This approach ensures that the colony as a whole will find an optimal path to a food source. Pheromone evaporation also helps ensure that if the current source runs out, or a closer one is found, the colony will continue to evolve to the globally optimal solution.
It’s a classic optimization solution that maximizes a critical outcome as efficiently as possible, and one that has been studied by entomologists, computer engineers and data scientists. In the current B2B marketing environment it can illuminate where we’re spending our time and money.
Beware the “Tyranny of the Mundane”
“It’s incredibly easy to get caught up in an activity trap, in the busy-ness of life, to work harder and harder at climbing the ladder of success only to discover it’s leaning against the wrong wall.
– Stephen Covey
The ants aren’t optimizing for the number of steps they take. Sales reps aren’t paid on the number of calls they make and real estate agents don’t get commission on the number of showings they do. Activity does not equate to outcome, and conflating the two can have really expensive implications.
The same story applies to marketers. We seem to spend a lot of effort fostering cultures of activity rather than outcomes.
A quick Google search for “Culture of Experimentation” reveals over 28 million results. These include innumerable guides from creating this culture within your organization to much more grand pronouncements like A culture of experimentation is the key to survival. The well respected consultants at McKinsey tell us that personalization is digital marketing’s “Holy Grail” while at the same time personalization is not what you think it is.
Experimentation, personalization, segmentation – these are activities and not outcomes. Blindly doing any one of these things without an understanding of how they contribute the end goal risks the dreaded activity trap.
Optimization brings focus on the outcome. Let’s define it for our purposes:
Marketing optimization is the process of improving the customer experience in an effort to maximize desired business outcomes.
Vendors talk about “website optimization” or “ad optimization” but in reality it’s the outcome that matters. Website optimization is simply the process of maximizing an outcome using website experiences.
Having a clear understanding of what’s necessary to optimize our end goal as efficiently as possible is essential to escaping the activity trap.
All outcomes aren’t created the same
It’s essential to make sure we’re optimizing for the right end-goal, the KPIs that ultimately moves the business forward. Of course all outcomes aren’t the same and if you’re not optimizing for the right goal you could waste a lot of time and effort.
In our A/B testing days we had a B2B client come to us that had spend well into the six figures on testing tools and teams. They had followed best practices and had plenty of “winning” tests measured by onsite conversion. Six months later they tried to assess the revenue impact of these winning tests and couldn’t find any.
This is a cautionary tale, however one that’s relatively common.
1/ OK, this is an infuriating startup experience: You ship an experiment that's +10% in your conversion funnel. Then your revenue/installs/whatever goes up by +10% right? Wrong 🙁 Turns out usually it goes up a little bit, or maybe not at all. Why is that?
— Andrew Chen (@andrewchen) May 3, 2018
Andrew Chen attributes this effect to what he calls “Conservation of Intent”. Most of your prospects have some intent to purchase (commercial intent). They don’t all have the same intent of course, but are instead distributed across a wide spectrum from low to high intent.
B2B marketers commonly run randomized experiments on intermediary goals such as website engagement, email opens and click through rates. These “vanity metrics” can be improved through relatively simplistic tactical means, such as making a CTA more prominent, changing subject lines and updating ad copy.
What Andrew suggests (and is borne out by our experience) is that although these experiments may improve the vanity metrics they tend to have a disproportionately greater effect on your low intent prospects. With Conservation of Intent in place you therefore don’t see the same impact further down the funnel (where it matters).
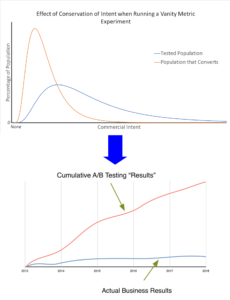
A winning vanity metric experiment will move more low intent prospects into the funnel. Over time the down funnel results are significantly worse than the accumulated “winners”.
You can think about the effect of Conservation of Intent as an efficiency penalty on your optimization effort. As the “distance” between the optimization goal and revenue increases, so does this penalty and the improvement you’ll have to achieve to affect revenue.
If you’re ultimately responsible for delivering pipeline or revenue and you’re optimizing for bounce rates and emails open, you’re going to have to work really hard and see huge increases in these goals. Lots of effort, lots of experiments and ultimately lots of money.
How do you avoid this activity trap? Optimize for the metrics that matter.
Optimization Efficiency – Striking a balance
Choosing the right outcomes is part of avoiding wasted effort, but there’s another consideration in understanding the efficiency of your optimization program. To do so it’s essential to understand its two necessary phases:
- Exploration – The process of learning about the relationship between context, an experience and the outcome. A/B tests and other experiments are examples of exploration.
- Exploitation – Maximizing outcomes based on previously established learnings. Hardcoding experiments and personalization are examples of exploitation.
I’ve found that a root cause of inefficient optimization is a poor understanding of the explore / exploit dynamic and how they need to work together to optimize outcomes. To better understand this let’s work through how we might traditionally optimize a landing page.
Our goal here is to increase form submissions from the landing page using traditional “manual” methods of experimentation and exploitation.
Exploration through randomized experimentation
When you run any kind of experiment you’re trying to learn something. In fact, you’re making a conscious decision to prioritize learnings over outcomes in the context of that experiment.
As marketers we’re often trying to learn about our customers and how they respond to certain experiences as measured by outcomes. Specifically what we’re trying to establish is a relationship (correlation) between something that we’re changing (known as the independent variable) and something that may be affected by it (the outcome or dependent variable).
You may never have thought of it this way but a well constructed hypothesis makes an assertion about the relationship between an independent and dependent variable.
For our landing page we might come up with the following hypothesis:
“If we change the headline to Save Money Today on my landing page I’ll see a 20% increase in form submissions because it’s a stronger benefit for visitors”.
There are five key elements of this hypothesis to understand:
- “change the headline” – The independent variable, what we’re going to change to to evaluate the effect on the outcome or dependent variable.
- “20% increase” – A statement about the expected relationship or correlation between the independent variable and the outcome.
- “form submissions” – The dependent variable or outcome.
- “because it’s a stronger benefit” – A presumed cause of the relationship between the variables.
- “visitors” – The customer population that we’re trying learn about, in this case visitors to the landing page.
Testing platforms make it easy to run experiments. But a common cause for false or misleading learnings is doing so in the absence of hypotheses which considers the above elements as well as an appropriately designed experiment that isolates the relationship between variables.
The experiment itself is not the end goal of exploration but a way to gather empirical evidence about the hypothesis, or relationship between the outcome and the independent variable. With randomized experimentation we’re going to send some percentage of the traffic to the baseline (control) experience and the rest to the new experiences (variations).
It’s important to note that by running this experiment we’re inevitably going to send some visitors to the “wrong” experience (one where they’re less likely to convert). We’re ok with that though – exploration maximizing learnings about the correlation in the present so we can maximize outcome in the future.
A quick sidebar on statistical significance. The whole reason you’re gathering this evidence is to inform a decision that you’re going make in the future. The (relatively poorly understood) role of statistical significance is to provide context about the evidence that you’ve gathered in the hope of mitigating what could be a expensive “wrong” decision.
If we run the experiment and don’t get our 20% increase we’ll probably reject or set aside this hypothesis and move on to another one. But let’s say we beat our expectations and see a 30% increase in form submissions and are confident that they’re not due to chance, and we can extrapolate the results to the general population. What does that mean?
Unless we take advantage of the observed correlation the experiment itself has little long term economic benefit – that’s the exploitation phase.
As marketers we’re probably also going to ask why we saw the results that we saw and what that might tell us about our customers. This causal inference actually has no bearing on the either this specific correlation or our ability to maximize outcomes from it – although it is certainly very important for understanding our customers and developing future hypotheses.
Exploitation through manual methods
If from our exploration we’ve observed positive correlations that we think have a meaningful business impact we shift to the other phase of optimization – exploitation. With exploitation we’re no longer prioritizing learnings, we’re trying to maximize the impact of previously identified ones. Running a lot of experiments without efficiently exploiting is an expensive proposition – especially in the absence of meaningful outcomes.
In our landing page example it’s a relatively straightforward process. Since the population for the experiment was all visitors to the landing page we’re going to change or “hardcode” the variation as the new baseline and we’re done.
Other channels are slightly more automated – most of today’s email tools will automatically send the winning variation once they’ve gathered evidence on a small sample.
Our initial hypothesis was (absurdly) simple. What if instead we changed it to:
“If we change the headline to Attend our VIP Event to decision makers at our target accounts who have seen a demo we’ll realize a 20% increase in opportunities generated because it’s a more relevant experience for this valuable, high intent audience”.
Let’s say you could actually run an experiment for this very specific group that measured opportunities (no small feat) and you achieve the expected results. Exploitation becomes significantly more complex since you can’t just updated the page with this headline and serve it to everyone. You need the relevant context about the visitor in order to correctly target them.
As marketers we know that delivering more relevant, personalized experiences based on context can be extremely valuable. Although often presented as a stand alone practice personalization is really just another method of exploitation. To optimize outcomes for these fine grained audiences therefore means that our personalization efforts must be informed by equivalently fine grained exploration.
The traditional approach to personalization requires the use of manually defined segments or audiences and are often very resource intensive to to set up and manage. If you’re investing in setting up segments with a-priori assumptions (not doing the relevant exploration) you will likely not be able to justify that investment.
Where does manual optimization fall short?
The viability of any marketing effort must be determined based on Return on Investment. If we can increase the theoretical return as well as the efficiency of our optimization efforts we will maximize ROI.
Manual optimization as described involves the use of randomized experimentation and segmentation. They rely on humans to make decisions and set up rules and consequently suffer from some significant barriers to achieving ROI, especially in B2B environments.
Measuring Vanity Metrics
Form fills, ad clicks and email opens. Manual experimentation requires that we gather sufficient evidence within a certain amount of time in order to minimize loss and maximize outcome. But as we’ve seen the further away you measure from revenue the less efficient your optimization efforts will be.
Precision
Most randomized experimentation use cases (like our landing page) are one-size-fits-all approaches. We’re trying to identify the single highest performing variation for an entire population. But what happens if that population is very diverse and sub-populations prefer (convert better) on different variations?
Trying to identify a single, global “winner” for an “average” customer that doesn’t exist results in ineffectual hypotheses and wasted experiences that have no long term utility. If a variation performs better for a small subset of your population but not good enough to be the global winner you end up throwing it out.
Even if you did create rules to identify those sub-populations there’s a limit to how granular (precise) you can be based on the effort required.
Investment
The more complex your customer environment is, the harder rules-based personalization becomes. Although there is considerable return in personalization in B2B markets, the investment required scales as a power of the number of customer dimensions and rapidly outpaces the potential return.
Number of Correlations
In a manual experiment with a single hypothesis you can establish one correlation at a time. Then you wait to gather sufficient evidence to be confident in the observed results because you have to make a non-trivial cost decision on how to proceed. Increasing the number of relationships you can establish in a period of time will increase the number you can exploit for outcome.
Exploration to Exploitation Frequency and Cost
Marketers that experiment more tend to outperform their competitors. While this is true, the root cause is not solely that they are experimenting more. To understand this take the extreme case – if you ran 10,000 experiments in a year but didn’t exploit any of them (move to 100%, hardcode or personalize) would you have maximized outcomes?
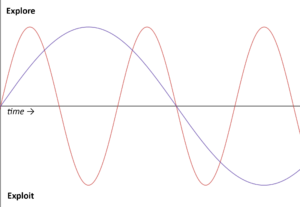
It’s not only about how fast you experiment. It’s about how fast you cycle between explore & exploit and the impact you have in each phase.
Rather than focusing on the frequency of experimentation, marketers that perform better tend to increase the frequency of both exploration & exploitation. There are limitations however to increasing this frequency with manual approaches, primarily related to the cost involved. If it requires a lot of effort (and therefore cost) to exploit a successful experiment, the frequency will be lower along with opportunities for success.
Bending the Curve with Autonomous Decisions
Not solving the systemic challenges with manual optimization means that we’ll continue to invest in increasingly low return approaches to optimization. The curve of diminishing returns is familiar to every marketer across every channel and in this case the requirement for manual decision making in optimization is a root cause.
How do we solve this? At FunnelEnvy our Predictive Revenue Optimization (PRO) platform is built on the vision of autonomous marketing decisions. Manual optimization lets us gather data and automate some processes, but the burden of interpreting that data and making decisions still falls on us. Autonomous optimization automates both processes and decisions.
Much like the shift from cruise control and parking assist to self-driving vehicles, autonomous decisioning in marketing will allow those who adopt it to achieve rapidly increasing returns.
Optimize for Revenue
B2B marketers are certainly interested in the impact they’re having on revenue, but largely rely on marketing attribution tools to assess it. Rather than producing channel-level reports that marketers have to act on, autonomous optimization integrates all available outcome data (including revenue) into progressively improving predictive decisions.
1:1 Experiences
As humans we require abstract groupings to reason about anything but the smallest populations populations (segments, MQLs, etc). A machine can evaluate context about individuals in the form of individual attributes from a variety of sources in real time. By correlating these individual attributes (of which there could be thousands or more) concurrently to outcomes we can achieve a level of precision that humans can’t.
Scale
The largest barrier to scaling personalized optimization using traditional tools is the investment required to create and manage rules-based segments. By reducing or eliminating the need for them you enable highly effective & personalized experiences at scale in even the most complex environments.
Continuous Optimization
Autonomous optimization doesn’t rely on fixed exploration and exploitation phases. Those concepts are still very relevant, however they increase the frequency of the two to their theoretical limit, with every impression or experience delivered. By dynamically tuning the ratio of the two they’ll be able to favor learnings or outcomes at any point in time.
Where do you fit into this world?
You’re either in a dystopian panic right now or planning an extended vacation to let the robots do all the work. But even in this age of AI computers are limited in terms of what they can do, and don’t truly “learn” the way we do. Marketers are still going to be required, but in an autonomous world will focus on the more impactful activities.
Marketers will still have to create the experiences to engage and convert customers. We’ll still need to set constraints and intervene on the machine’s decisions to enforce business constraints where the cost of being wrong is still considerable. Finally, machines may be able to calculate correlations much faster than us, but the very important need to reason about why and truly learn about our customers can not be replaced.